《Editor’s Note: Students in the School of Urban and Environmental Engineering at UNIST are continuously making academic progress. Here is a brief summary of those inspiring stories.》
■ Dr. Fritz Sihombing, Appointed as Faculty Member of Calvin Institute of Technology (CIT)
Dr. Fritz Sihombing, who recently completed his Ph.D. in the School of Urban and Environmental Engineering at UNIST, has recently been appointed as an assistant professor in the Department of Structural and Geotechnical Engineering at Calvin Institute of Technology (CIT), located in Jakarta, Indonesia. His term will begin in December, 2019.
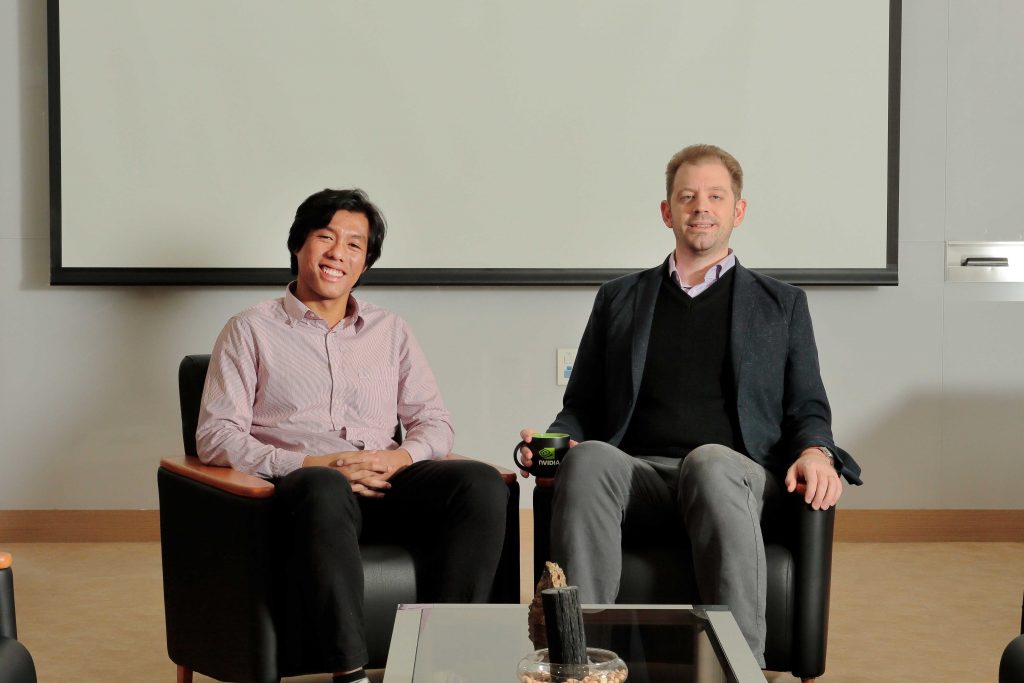
From left are Fritz Sihombing and his advisor, Professor Marco Torbol in the School of Urban and Environmental Engineering at UNIST.
“I am looking forward to teaching and to sharing my own experience and expertise in field of structural and geotechnical engineering at a place that fosters the ability of young scientists to independently undertake research projects and do,” says Dr. Sihombing. “This success is largely due to my past work of earthquakes and tsunami mitigation at UNIST.”
■ Two UNIST Students Honored by the Korea Meteorological Administration
Two students from a research lab, led by Professor Jungho Im in the School of Urban and Environmental Engineering at UNIST, have been recognized for research excellence at the ‘2019 Student Competition in Research, Using Meteorological Satellite’, held on November 12, 2019.
Organized by the Korea Meteorological Administration (KMA), the competition offers a great opportunity for students to demonstrate their knowledge of data science and satellite data analytics by competing with other students across the nation. For that, the competition focused on the development of algorithms, using satellite data, weather prediction system, and technologies related to applied climatology.
Among many prominent participants, Cheolhee Yoo (Graduate School of Urban and Environmental Engineering, UNIST) was highly praised for his research work on land surface temperature, thus was given the KMA Director Award, the highest accolade of the competition.
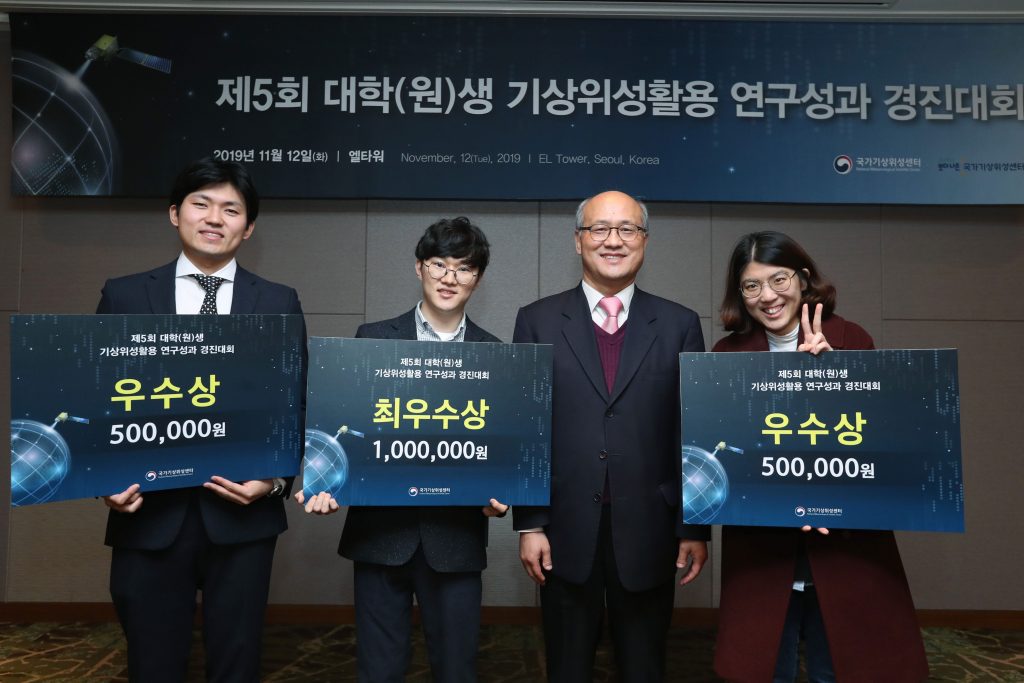
Cheolhee Yoo (Graduate School of Urban and Environmental Engineering, UNIST) was given the grand prize by the Korea Meteorological Administration. l Image Credit: Cheolhee Yoo
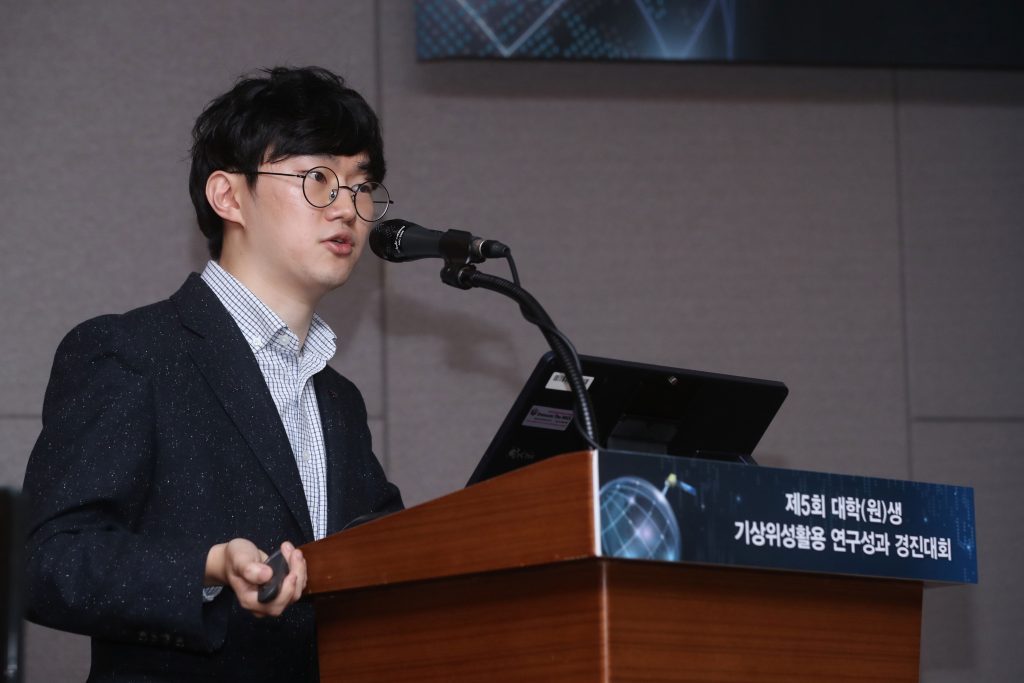
Cheolhee Yoo (Graduate School of Urban and Environmental Engineering, UNIST) was given the grand prize by the Korea Meteorological Administration. l Image Credit: Cheolhee Yoo
This award has been bestowed to Cheolhee Yoo in recognition of his research and development project for estimating the land surface temperatures under cloudy skies of Korean Peninsula, using fusion techniques for satellite images. The land surface temperature records, measured by satellites, have a key role in supporting the weather forecasts and climate-related research. However, it is difficult to monitor changes in surface temperature under cloudy conditions. Besides, when the moisture content in the air is especially high in the summer months, it becomes even harder to estimate. To solve this problem, Cheolhee Yoo has introduced a machine-learning approach for forecasting daytime and nighttime land surface temperatures.
“With this model, it is possible to analyze the detailed spatial and temporal characteristics of variations in surface air temperature over the Korean peninsula,” says Cheolhee Yoo. “It would be of great help for urban-climate studies related to heat island, as well as for dealing with natural disasters, like heat waves and droughts.”
Prior to this, Jaese Lee (Graduate School of Urban and Environmental Engineering, UNIST) from the same laboratory, also won the Best Paper Award (KAGIS President Award) at the 2019 Korean Association of Geographic Information Studies (KAGIS) Annual Fall Meeting. Held at Jeju National University (JNU) from October 31 to November 2, the conference dealt with issues, relating to the application of geographical information system, such as spatial analysis, remote sensing, and land planning.
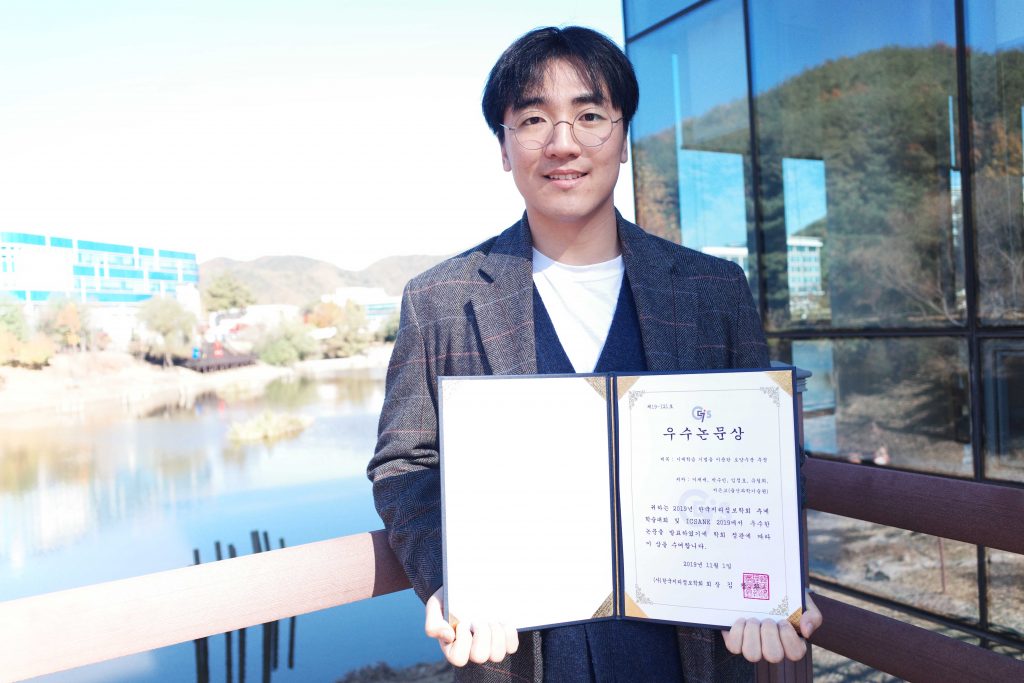
Jaese Lee (Graduate School of Urban and Environmental Engineering, UNIST) won the Best Paper Award at the 2019 Korean Association of Geographic Information Studies Annual Fall Meeting on Friday, November 1.
This award has been bestowed to Jaese Lee in recognition of his research and development project for estimating soil moisture content via satellite data. Accurate and timely information on soil moisture is critical for research in agriculture, climate modeling, and weather forecasts, as it has been difficult to get a complete picture based on the observed data.
Efforts have been made to improve soil moisture data management and delivery systems and Jaese Lee ‘s research was also part of it. Through this project, Jaese Lee proposed a method, capable of providing accurate readings of soil moisture content, obtained from satellites, using machine learning.