Woongki Baek, Professor in the Department of Computer Science and Engineering at UNIST has been selected as a recipient of Most Influential PLDI Paper Award at the 41st ACM SIGPLAN Conference on Programming Language Design and Implementation (PLDI 2020), which took place in London, UK, Wednesday, 17 June through Friday, 19 June 2020.
The award was presented in recognition of Professor Baek’s research paper, entitled “Green: A Framework for Supporting Energy-Conscious Programming using Controlled Approximation,” which was published in PLDI 2010. The award-winning paper by Professor Woongki Baek deals with the development of programming framework in support of approximate computing. Meanwhile, the PLDI (ACM SIGPLAN Conference on Programming Language Design and Implementation) is the top conference in the field of programming languages and compilers.
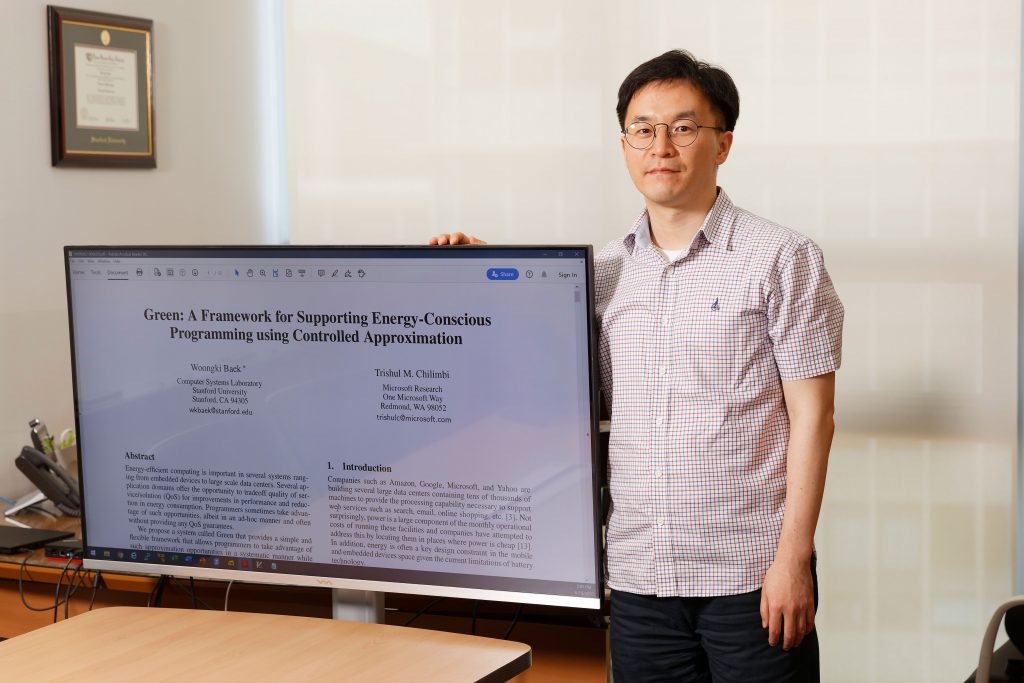
In recognition of his hard work, both academically and socioeconomically, Professor Baek was given the Most Influential PLDI Paper Award at PLDI 2020. l Image Credit: Kyoungchae Kim
“In general, computers are thought to carry out calculations and information processing with 100% accuracy. However, in various computing fields, such as image processing, machine learning, and web search, there is a high probability of obtaining the desired result even if there is loss-of-accuracy issue,” says Professor Baek. “Approximate computing technique can be applied to these fields to achieve a desired result, while reducing the cost and energy consumption required to process massive data sets.”
Most Influential PLDI Paper Award is presented annually to the author(s) of a paper presented at the PLDI held 10 years prior to the award year. These papers are, then, judged by their influence over the past decade. The selection committee for the Most Influential PLDI Paper Award cited the paper as follows.
“The PLDI paper “Green: A Framework for Supporting Energy-Conscious Programming using Controlled Approximation” by Woongki Baek and Trishul Chilimbi demonstrated conclusively two important things: (1) that frameworks supporting approximations that trade energy/performance costs for quality of service could be generalized and automated, and (2) applying this approach in data center search workloads could result in significant energy reduction and cost, saving millions of dollars in practice. Green proved that systematic approaches to approximate computing could have enormous commercial impact and predated and informed many subsequent machine learning optimizations, such as using reduced precision arithmetic in deep learning models. Green also inspired subsequent efforts, to more deeply encode approximation semantics into the underlying programming language design.”