An innovative artificial intelligence (AI) model, capable of predicting changes in Arctic sea ice up to a year in advance has been developed. This model is expected to provide invaluable mid- to long-term forecasting information, thereby assisting in the development of Arctic sea routes and the exploration of marine resources.
Professor Jungho Im from the Department of Civil, Urban, Earth, and Environmental Engineering at UNIST has created an AI model that predicts Arctic sea ice concentration for up to one year with an impressive accuracy of less than 6 percent error. Sea ice concentration refers to the proportion of a specific area that is covered by ice.
The research team employed the UNET deep learning algorithm to develop this model, which effectively learns the complex relationships between historical changes in Arctic sea ice concentration and key climatic factors, including air temperature, water temperature, solar radiation, and wind. UNET is a sophisticated deep learning framework that enables AI to analyze and understand relationships within image data, such as satellite imagery.
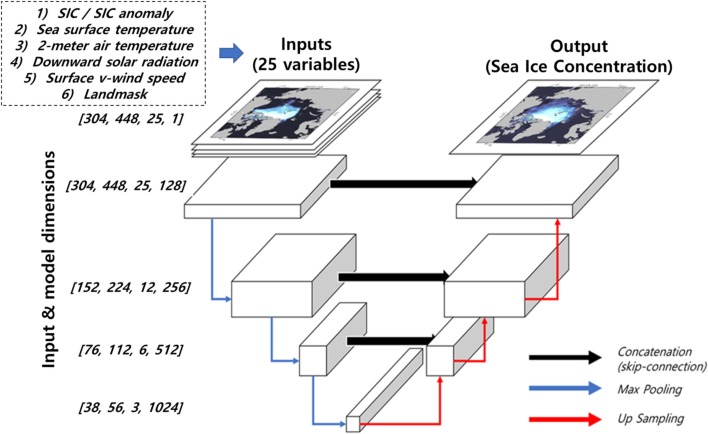
Figure 1. Schematic description of the UNET model used in this study.
The model demonstrated high accuracy in mid- to long-term forecasting. Through a rigorous evaluation comparing the AI model’s predictions against actual historical sea ice concentration values, the research team recorded an average prediction error of less than 6% for forecasts made at 3, 6, and 12 months. In contrast, existing models showed increasing error rates as the forecast period extended.
Moreover, the AI model exhibited stable predictive performance, even during periods of rapid sea ice decline. During significant melt seasons, such as the summers of 2007 and 2012, the new model maintained an average prediction error of 7.07%, substantially reducing the average prediction error by more than half compared to existing models, which averaged 17.35%.
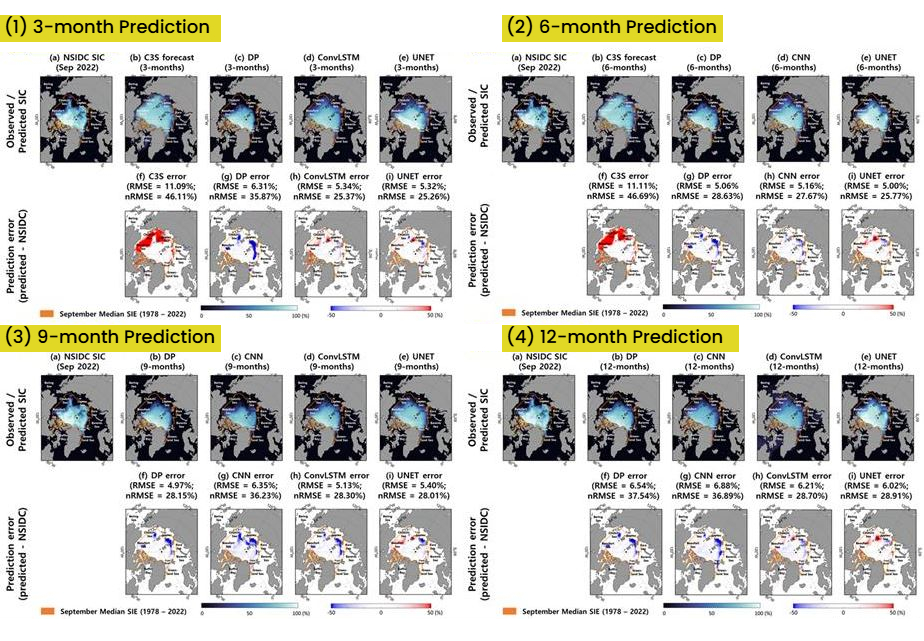
Figure 2. NSIDC sea ice concentrations.
The research team also identified critical climatic factors influencing mid- to long-term sea ice concentration predictions. Analysis of the UNET model’s outcomes revealed that solar radiation and wind are especially influential in areas where ice is thin.
Professor Im stated, “This study addresses the limitations of traditional physics-based models by exploring the complex interplay of various environmental factors that impact changes in Arctic sea ice.” He further emphasized that the findings will aid in the development of Arctic navigation routes, the exploration of marine resources, and the formulation of policies to address climate change.
Their findings have been published in the online version of Remote Sensing of Environment on December 11, 2024. It has received support from the Korea Polar Research Institute (KOPRI) and the Ministry of Oceans and Fisheries.
Journal Reference
Young Jun Kim, Hyun-cheol Kim, Daehyeon Han, et al., “Long-term prediction of Arctic sea ice concentrations using deep learning: Effects of surface temperature, radiation, and wind conditions,” Remote Sens. Environ., (2024).